Exercise V: Regularization¶
This exercise is an adaptation of lab 2 from the ISLR, in which the salary of baseball players is predicted on the basis of various statistics associated with performance in the previous year.
Set up¶
import pandas as pd
URL = "https://raw.githubusercontent.com/JWarmenhoven/ISLR-python/master/Notebooks/Data/Hitters.csv"
data = pd.read_csv(URL, index_col=0)
General Inspection and Preprocessing¶
data
AtBat | Hits | HmRun | Runs | RBI | Walks | Years | CAtBat | CHits | CHmRun | CRuns | CRBI | CWalks | League | Division | PutOuts | Assists | Errors | Salary | NewLeague | |
---|---|---|---|---|---|---|---|---|---|---|---|---|---|---|---|---|---|---|---|---|
-Andy Allanson | 293 | 66 | 1 | 30 | 29 | 14 | 1 | 293 | 66 | 1 | 30 | 29 | 14 | A | E | 446 | 33 | 20 | NaN | A |
-Alan Ashby | 315 | 81 | 7 | 24 | 38 | 39 | 14 | 3449 | 835 | 69 | 321 | 414 | 375 | N | W | 632 | 43 | 10 | 475.0 | N |
-Alvin Davis | 479 | 130 | 18 | 66 | 72 | 76 | 3 | 1624 | 457 | 63 | 224 | 266 | 263 | A | W | 880 | 82 | 14 | 480.0 | A |
-Andre Dawson | 496 | 141 | 20 | 65 | 78 | 37 | 11 | 5628 | 1575 | 225 | 828 | 838 | 354 | N | E | 200 | 11 | 3 | 500.0 | N |
-Andres Galarraga | 321 | 87 | 10 | 39 | 42 | 30 | 2 | 396 | 101 | 12 | 48 | 46 | 33 | N | E | 805 | 40 | 4 | 91.5 | N |
... | ... | ... | ... | ... | ... | ... | ... | ... | ... | ... | ... | ... | ... | ... | ... | ... | ... | ... | ... | ... |
-Willie McGee | 497 | 127 | 7 | 65 | 48 | 37 | 5 | 2703 | 806 | 32 | 379 | 311 | 138 | N | E | 325 | 9 | 3 | 700.0 | N |
-Willie Randolph | 492 | 136 | 5 | 76 | 50 | 94 | 12 | 5511 | 1511 | 39 | 897 | 451 | 875 | A | E | 313 | 381 | 20 | 875.0 | A |
-Wayne Tolleson | 475 | 126 | 3 | 61 | 43 | 52 | 6 | 1700 | 433 | 7 | 217 | 93 | 146 | A | W | 37 | 113 | 7 | 385.0 | A |
-Willie Upshaw | 573 | 144 | 9 | 85 | 60 | 78 | 8 | 3198 | 857 | 97 | 470 | 420 | 332 | A | E | 1314 | 131 | 12 | 960.0 | A |
-Willie Wilson | 631 | 170 | 9 | 77 | 44 | 31 | 11 | 4908 | 1457 | 30 | 775 | 357 | 249 | A | W | 408 | 4 | 3 | 1000.0 | A |
322 rows × 20 columns
Well, it looks like a dataset.
Let’s probe a little deeper:
data.info()
<class 'pandas.core.frame.DataFrame'>
Index: 322 entries, -Andy Allanson to -Willie Wilson
Data columns (total 20 columns):
# Column Non-Null Count Dtype
--- ------ -------------- -----
0 AtBat 322 non-null int64
1 Hits 322 non-null int64
2 HmRun 322 non-null int64
3 Runs 322 non-null int64
4 RBI 322 non-null int64
5 Walks 322 non-null int64
6 Years 322 non-null int64
7 CAtBat 322 non-null int64
8 CHits 322 non-null int64
9 CHmRun 322 non-null int64
10 CRuns 322 non-null int64
11 CRBI 322 non-null int64
12 CWalks 322 non-null int64
13 League 322 non-null object
14 Division 322 non-null object
15 PutOuts 322 non-null int64
16 Assists 322 non-null int64
17 Errors 322 non-null int64
18 Salary 263 non-null float64
19 NewLeague 322 non-null object
dtypes: float64(1), int64(16), object(3)
memory usage: 52.8+ KB
Calling info()
reveals that most (16/19) columns contain integers, except for Salary
which is a float
and League
, NewLeague
, and Division
which are object
(categorical). Even more importantly, it shows that Salary
contains missing observations. Because Salary
is our target variable, we don’t really have any use for these observations and would be better off simply dropping them from our dataset:
data.dropna(subset=["Salary"], inplace=True)
Done.
Next, we might give describe()
a quick look:
pd.set_option('float_format', '{:.4g}'.format)
data.describe()
AtBat | Hits | HmRun | Runs | RBI | Walks | Years | CAtBat | CHits | CHmRun | CRuns | CRBI | CWalks | PutOuts | Assists | Errors | Salary | |
---|---|---|---|---|---|---|---|---|---|---|---|---|---|---|---|---|---|
count | 263 | 263 | 263 | 263 | 263 | 263 | 263 | 263 | 263 | 263 | 263 | 263 | 263 | 263 | 263 | 263 | 263 |
mean | 403.6 | 107.8 | 11.62 | 54.75 | 51.49 | 41.11 | 7.312 | 2658 | 722.2 | 69.24 | 361.2 | 330.4 | 260.3 | 290.7 | 118.8 | 8.593 | 535.9 |
std | 147.3 | 45.13 | 8.757 | 25.54 | 25.88 | 21.72 | 4.794 | 2287 | 648.2 | 82.2 | 331.2 | 323.4 | 264.1 | 279.9 | 145.1 | 6.607 | 451.1 |
min | 19 | 1 | 0 | 0 | 0 | 0 | 1 | 19 | 4 | 0 | 2 | 3 | 1 | 0 | 0 | 0 | 67.5 |
25% | 282.5 | 71.5 | 5 | 33.5 | 30 | 23 | 4 | 842.5 | 212 | 15 | 105.5 | 95 | 71 | 113.5 | 8 | 3 | 190 |
50% | 413 | 103 | 9 | 52 | 47 | 37 | 6 | 1931 | 516 | 40 | 250 | 230 | 174 | 224 | 45 | 7 | 425 |
75% | 526 | 141.5 | 18 | 73 | 71 | 57 | 10 | 3890 | 1054 | 92.5 | 497.5 | 424.5 | 328.5 | 322.5 | 192 | 13 | 750 |
max | 687 | 238 | 40 | 130 | 121 | 105 | 24 | 1.405e+04 | 4256 | 548 | 2165 | 1659 | 1566 | 1377 | 492 | 32 | 2460 |
Scaling¶
I seems like there’s fairly large variabiliy in scale between the different features, and in any case regularization requires some form of standardization.
First, let’s differntiate the features matrix (X
) from the target vector (y
):
target_name = "Salary"
X = data.drop(columns=[target_name])
y = data[target_name].copy()
Only numeric columns should be standardized, so let’s also be sure to distinguish those from the categoricals:
numeric_features = X.select_dtypes([int, float])
And we’re all set:
from sklearn.preprocessing import StandardScaler
scaler = StandardScaler()
X.loc[:, numeric_features.columns] = scaler.fit_transform(
X.loc[:, numeric_features.columns])
X
AtBat | Hits | HmRun | Runs | RBI | Walks | Years | CAtBat | CHits | CHmRun | CRuns | CRBI | CWalks | League | Division | PutOuts | Assists | Errors | NewLeague | |
---|---|---|---|---|---|---|---|---|---|---|---|---|---|---|---|---|---|---|---|
-Alan Ashby | -0.6029 | -0.5957 | -0.5286 | -1.206 | -0.5221 | -0.09753 | 1.398 | 0.3468 | 0.1744 | -0.00292 | -0.1217 | 0.259 | 0.4353 | N | W | 1.221 | -0.5232 | 0.2134 | N |
-Alvin Davis | 0.5125 | 0.4923 | 0.73 | 0.4415 | 0.7941 | 1.609 | -0.9012 | -0.4529 | -0.4099 | -0.07605 | -0.4151 | -0.1996 | 0.01037 | A | W | 2.109 | -0.2539 | 0.82 | A |
-Andre Dawson | 0.6282 | 0.7365 | 0.9588 | 0.4023 | 1.026 | -0.1898 | 0.7709 | 1.302 | 1.318 | 1.899 | 1.412 | 1.573 | 0.3557 | N | E | -0.3247 | -0.7442 | -0.8482 | N |
-Andres Galarraga | -0.5621 | -0.4625 | -0.1853 | -0.6177 | -0.3672 | -0.5127 | -1.11 | -0.9909 | -0.9602 | -0.6977 | -0.9475 | -0.8812 | -0.8623 | N | E | 1.841 | -0.5439 | -0.6966 | N |
-Alfredo Griffin | 1.295 | 1.358 | -0.8718 | 0.7553 | -0.01884 | -0.2821 | 0.7709 | 0.767 | 0.635 | -0.6124 | 0.4228 | 0.01729 | -0.2514 | A | W | -0.03118 | 2.087 | 2.488 | A |
... | ... | ... | ... | ... | ... | ... | ... | ... | ... | ... | ... | ... | ... | ... | ... | ... | ... | ... | ... |
-Willie McGee | 0.635 | 0.4257 | -0.5286 | 0.4023 | -0.135 | -0.1898 | -0.4832 | 0.01992 | 0.1295 | -0.4539 | 0.05378 | -0.06016 | -0.4639 | N | E | 0.1227 | -0.758 | -0.8482 | N |
-Willie Randolph | 0.601 | 0.6255 | -0.7574 | 0.8338 | -0.05755 | 2.44 | 0.9799 | 1.25 | 1.219 | -0.3686 | 1.621 | 0.3736 | 2.332 | A | E | 0.07977 | 1.811 | 1.73 | A |
-Wayne Tolleson | 0.4853 | 0.4034 | -0.9862 | 0.2454 | -0.3285 | 0.5022 | -0.2742 | -0.4196 | -0.447 | -0.7586 | -0.4363 | -0.7356 | -0.4336 | A | W | -0.9081 | -0.03978 | -0.2416 | A |
-Willie Upshaw | 1.152 | 0.8031 | -0.2997 | 1.187 | 0.3295 | 1.702 | 0.1438 | 0.2368 | 0.2084 | 0.3384 | 0.3291 | 0.2776 | 0.2722 | A | E | 3.662 | 0.08452 | 0.5167 | A |
-Willie Wilson | 1.546 | 1.38 | -0.2997 | 0.873 | -0.2898 | -0.4666 | 0.7709 | 0.9861 | 1.136 | -0.4783 | 1.252 | 0.08236 | -0.04275 | A | W | 0.4198 | -0.7925 | -0.8482 | A |
263 rows × 19 columns
X.describe()
AtBat | Hits | HmRun | Runs | RBI | Walks | Years | CAtBat | CHits | CHmRun | CRuns | CRBI | CWalks | PutOuts | Assists | Errors | |
---|---|---|---|---|---|---|---|---|---|---|---|---|---|---|---|---|
count | 263 | 263 | 263 | 263 | 263 | 263 | 263 | 263 | 263 | 263 | 263 | 263 | 263 | 263 | 263 | 263 |
mean | 1.013e-17 | 5.741e-17 | 3.377e-17 | -5.066e-17 | 1.216e-16 | 1.689e-18 | -5.403e-17 | 6.079e-17 | 6.754e-17 | 5.403e-17 | 3.377e-17 | 4.053e-17 | 1.081e-16 | 7.43e-17 | 0 | 1.047e-16 |
std | 1.002 | 1.002 | 1.002 | 1.002 | 1.002 | 1.002 | 1.002 | 1.002 | 1.002 | 1.002 | 1.002 | 1.002 | 1.002 | 1.002 | 1.002 | 1.002 |
min | -2.616 | -2.372 | -1.329 | -2.148 | -1.993 | -1.897 | -1.319 | -1.156 | -1.11 | -0.844 | -1.087 | -1.014 | -0.9837 | -1.04 | -0.8201 | -1.303 |
25% | -0.8239 | -0.8066 | -0.7574 | -0.8334 | -0.8317 | -0.8356 | -0.6922 | -0.7953 | -0.7886 | -0.6611 | -0.7736 | -0.7294 | -0.7181 | -0.6343 | -0.7649 | -0.8482 |
50% | 0.06364 | -0.1072 | -0.2997 | -0.1077 | -0.1737 | -0.1898 | -0.2742 | -0.3183 | -0.3187 | -0.3564 | -0.3365 | -0.3111 | -0.3273 | -0.2388 | -0.5094 | -0.2416 |
75% | 0.8322 | 0.7476 | 0.73 | 0.7161 | 0.7554 | 0.7329 | 0.5619 | 0.5402 | 0.5129 | 0.2835 | 0.4123 | 0.2915 | 0.2589 | 0.1138 | 0.5058 | 0.6683 |
max | 1.927 | 2.89 | 3.247 | 2.952 | 2.691 | 2.947 | 3.488 | 4.993 | 5.462 | 5.836 | 5.457 | 4.116 | 4.954 | 3.888 | 2.578 | 3.55 |
Warning
Try to consider the way in which standardization was applied. Can you think of anything that could pose a problem in following steps?
Solution
Applying standardization prior to the train-test split results in information (mean and standard deviation) from the test set “leaking” into the training set, and therefore should be avoided! The correct procedure is to apply standardization separately after splitting the data.
Dummy Encoding¶
To view the different values each categorical variable holds:
for feature in X.select_dtypes(object):
print(feature.ljust(10), X[feature].unique())
League ['N' 'A']
Division ['W' 'E']
NewLeague ['N' 'A']
And to “dummy encode” it is as simple as:
X = pd.get_dummies(X, drop_first=True)
X.sample(20).select_dtypes(include=["uint8"]).style.background_gradient()
League_N | Division_W | NewLeague_N | |
---|---|---|---|
-Ken Landreaux | 1 | 1 | 1 |
-Ron Hassey | 0 | 0 | 0 |
-Shawon Dunston | 1 | 0 | 1 |
-Chris Bando | 0 | 0 | 0 |
-Don Mattingly | 0 | 0 | 0 |
-Ken Phelps | 0 | 1 | 0 |
-Gary Carter | 1 | 0 | 1 |
-Al Newman | 1 | 0 | 0 |
-Rick Dempsey | 0 | 0 | 0 |
-Wally Joyner | 0 | 1 | 0 |
-Kevin Bass | 1 | 1 | 1 |
-Leon Durham | 1 | 0 | 1 |
-Jesse Barfield | 0 | 0 | 0 |
-Brian Downing | 0 | 1 | 0 |
-Tom Brunansky | 0 | 1 | 0 |
-Terry Kennedy | 1 | 1 | 0 |
-Jody Davis | 1 | 0 | 1 |
-Dwight Evans | 0 | 0 | 0 |
-Argenis Salazar | 0 | 1 | 0 |
-Ted Simmons | 1 | 1 | 1 |
Model Creation¶
In the previous linear regression exercise we used sklearn
’s LinearRegression
class along with the train_test_split()
function to model the relationship between the features and the target variable and evaluate it’s performance in predicting the target variable for an out-of-sample collection of observations. In this exercise, we will use LassoCV
and RidgeCV
to introduce the \(\ell_1\) and \(\ell_2\) penalties as part of our fitting process and regularize the coefficients of our predictors. In addition, cross-validation will also be taken care of automatically.
For the sake of uniformity we’ll use the same list of regularization parameter values:
import numpy as np
alphas = np.linspace(0.01, 100, 200)
Lasso (\(\ell_1\))
from sklearn.linear_model import LassoCV
lasso_model = LassoCV(alphas=alphas, max_iter=10000)
_ = lasso_model.fit(X, y)
Ridge (\(\ell_2\))
from sklearn.linear_model import RidgeCV
ridge_model = RidgeCV(alphas=alphas, store_cv_values=True)
_ = ridge_model.fit(X, y)
Note
By default, LassoCV
will use 5-fold cross-validation with \(R^2\) as the scoring method and RidgeCV
will use Generalized Cross-Validation, which is a form of efficient Leave-One-Out cross-validation, with negative mean squared error.
To change the splitting strategy, set the cv
keyword argument to the number of folds you’d like to use, to specify scoring and other search parameters see the GridSearchCV
class.
Model Application¶
To calculate the predicted salary of each player using the model:
Lasso (\(\ell_1\))
lasso_predictions = lasso_model.predict(X)
Ridge (\(\ell_2\))
ridge_predictions = ridge_model.predict(X)
Model Evaluation¶
import matplotlib.pyplot as plt
def plot_predictions(y: pd.Series, predictions: pd.Series, ax: plt.Axes):
ax.scatter(y, predictions, color="black")
ax.plot(y, y, color="red")
ax.set_xlabel("Real Values")
ax.set_ylabel("Predicted Values")
fig, ax = plt.subplots(ncols=2, figsize=(12, 5))
plot_predictions(y, lasso_predictions, ax[0])
ax[0].set_title(r"Lasso ($\ell_1$)")
plot_predictions(y, ridge_predictions, ax[1])
_ = ax[1].set_title(r"Lasso ($\ell_2$)")
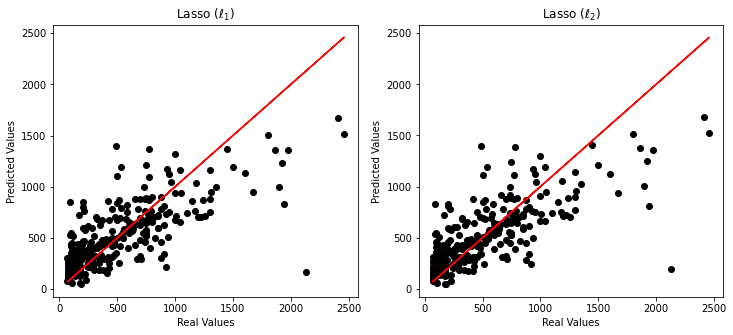
Alpha¶
Lasso (\(\ell_1\))
lasso_model.alpha_
2.522311557788944
Ridge (\(\ell_2\))
ridge_model.alpha_
3.527236180904522
Coefficients¶
coefficients_dict = {"Lasso": lasso_model.coef_, "Ridge": ridge_model.coef_}
coefficients = pd.DataFrame(coefficients_dict, index=X.columns)
coefficients.T
AtBat | Hits | HmRun | Runs | RBI | Walks | Years | CAtBat | CHits | CHmRun | CRuns | CRBI | CWalks | PutOuts | Assists | Errors | League_N | Division_W | NewLeague_N | |
---|---|---|---|---|---|---|---|---|---|---|---|---|---|---|---|---|---|---|---|
Lasso | -237.2 | 261.6 | 0 | -0 | 0 | 104.8 | -46.64 | -0 | 0 | 44.29 | 224.5 | 123.9 | -144.8 | 76.81 | 26.48 | -13.56 | 27.04 | -114.1 | 0 |
Ridge | -216.4 | 230.7 | 0.9447 | 0.7865 | 6.002 | 106.3 | -51.34 | -94.18 | 119.4 | 58.18 | 199.9 | 114.5 | -144.5 | 77.68 | 39.18 | -24.86 | 50.73 | -118.6 | -18.02 |
from sklearn.linear_model import Lasso, Ridge
coef_fig, coef_ax = plt.subplots(ncols=2, figsize=(14, 6))
#########
# Lasso #
#########
simple_lasso = Lasso(max_iter=10_000)
lasso_coefficient_values = []
lasso_alphas = lasso_model.alphas_[::-1]
for alpha in lasso_alphas:
simple_lasso.set_params(alpha=alpha)
simple_lasso.fit(X, y)
lasso_coefficient_values.append(simple_lasso.coef_)
# Coefficient values by alpha
coef_ax[0].semilogx(lasso_alphas, lasso_coefficient_values, linestyle="dashed", alpha=0.5)
# Chosen alpha
coef_ax[0].axvline(lasso_model.alpha_,
linestyle='--',
color="black",
label=r"Chosen $\alpha$")
coef_ax[0].legend()
coef_ax[0].set_xlabel(r'$\alpha$')
coef_ax[0].set_ylabel("Coefficient Values")
coef_ax[0].set_title(r"Lasso: Coefficient Values by $\alpha$")
#########
# Ridge #
#########
simple_ridge = Ridge()
ridge_coefficient_values = []
ridge_alphas = ridge_model.alphas[::-1]
for alpha in ridge_alphas:
simple_ridge.set_params(alpha=alpha)
simple_ridge.fit(X, y)
ridge_coefficient_values.append(simple_ridge.coef_)
# Coefficient values by alpha
coef_ax[1].semilogx(ridge_alphas,
ridge_coefficient_values,
linestyle="dashed",
alpha=0.5)
# Chosen alpha
coef_ax[1].axvline(ridge_model.alpha_,
linestyle='--',
color="black",
label=r"Chosen $\alpha$")
coef_ax[1].legend(X.columns, loc="upper left", bbox_to_anchor=(1.05, 0.95))
coef_ax[1].set_xlabel(r'$\alpha$')
coef_ax[1].set_ylabel("Coefficient Values")
_ = coef_ax[1].set_title(r"Ridge: Coefficient Values by $\alpha$")
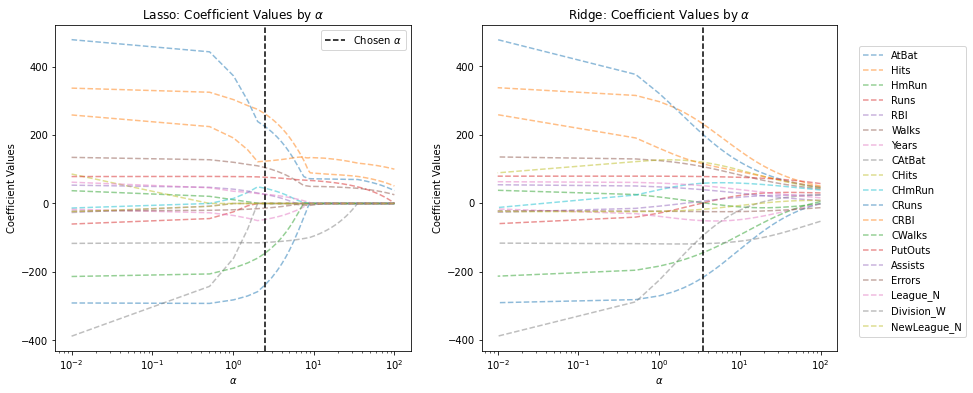
Mean Squared Error (MSE)¶
mse_fig, mse_ax = plt.subplots(ncols=2, figsize=(14, 6))
#########
# Lasso #
#########
lasso_alphas = lasso_model.alphas_
lasso_mse = lasso_model.mse_path_
# Cross-validation MSEs
mse_ax[0].semilogx(lasso_alphas,
lasso_mse,
linestyle="dashed",
color="blue",
alpha=0.2)
# Mean MSE
mse_ax[0].plot(lasso_alphas,
lasso_mse.mean(axis=-1),
color="black",
label="Mean MSE Across Folds",
linewidth=2)
# Chosen alpha
mse_ax[0].axvline(lasso_model.alpha_,
linestyle='--',
color="black",
label=r"Chosen $\alpha$")
mse_ax[0].legend()
mse_ax[0].set_xlabel(r'$\alpha$')
mse_ax[0].set_ylabel("MSE")
mse_ax[0].set_title("Lasso: Mean Squared Error (MSE) Across Folds")
#########
# Ridge #
#########
ridge_alphas = ridge_model.alphas
ridge_mse = ridge_model.cv_values_.T
# Cross-validation MSEs
mse_ax[1].semilogx(ridge_alphas,
ridge_mse,
linestyle="dashed",
color="blue",
alpha=0.2)
# Mean MSE
mse_ax[1].plot(ridge_alphas,
ridge_mse.mean(axis=-1),
color="black",
label="Mean MSE Across Folds",
linewidth=2)
# Chosen alpha
mse_ax[1].axvline(ridge_model.alpha_,
linestyle='--',
color="black",
label=r"Chosen $\alpha$")
mse_ax[1].legend()
mse_ax[1].set_xlabel(r'$\alpha$')
mse_ax[1].set_ylabel("MSE")
mse_ax[1].ticklabel_format(style="plain", axis="y")
mse_ax[1].set_ylim(mse_ax[0].get_ylim())
_ = mse_ax[1].set_title("Ridge: Mean Squared Error (MSE) Across Folds")
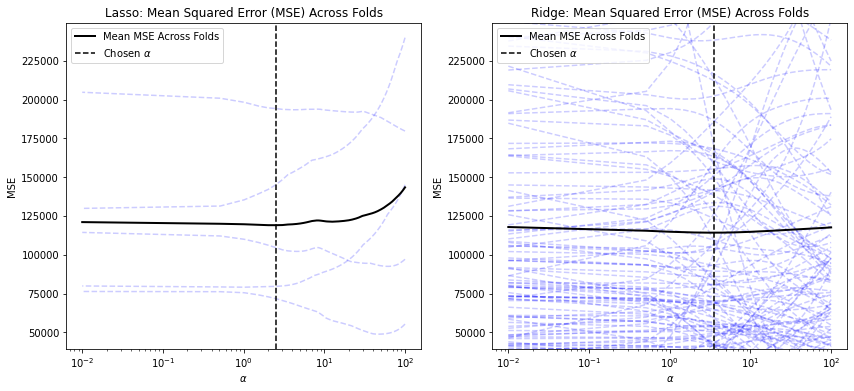
\(R^2\)¶
To calculate in-sample \(R^2\) score for each model:
Lasso (\(\ell_1\))
lasso_model.score(X, y)
0.5342574616741457
Ridge (\(\ell_2\))
ridge_model.score(X, y)
0.5364706351171298
Note that calculating in-sample \(R^2\) scores will result in underestimating prediction error.
Note
For a more generalized solution for parameter tuning, see sklearn
’s GridSearchCV
.